Northwestern University’s Data Science Initiative has its origin in a Lawrence B. Dumas Domain Dinner titled “The Possibilities of Big Data” that took place in May 2013. The Domain Dinner Program, which originated in 1998 as a result of the strategic planning process for The Highest Order of Excellence, the University’s strategic plan at the time, is designed to stimulate faculty interactions across departments and disciplines and highlight Northwestern’s distinctive culture of interdisciplinary collaboration.
Planning for the dinner was chaired by Luis Amaral and involved the participation representatives from a broad range of disciplines. The speakers, Cate Brinson (material scientist, Engineering), David Figlio (economist, Education and Social Policy), Michael Schmitt (physicist, Arts and Sciences), and Justin Starren (physician and informatician, Medicine), were joined during the Q&A session Vicky Kalogera (astronomy, Arts and Sciences) and Brian Uzzi (management and organizations, Management).
“The possibilities of Big Data” was one of the best attended Domain Dinners in the two decade history of the Program. The widespread interest prompted the organization of a half-day workshop aimed at increasing faculty know-how for pursuing Data Science research. The workshop aimed to establish inter-disciplinary relationships between faculty, teach faculty about resources at Northwestern, and help Northwestern’s Information Technologies group identify infrastructural needs for Data Science research.
The workshop, which took place in January 2014, was attended by over 100 faculty members from the Chicago and Evanston campuses. As the Domain Dinner, the workshop featured a diversity of set of presenters and participants representing pretty much every School at the University. The presenters included Kristian Hahn and Vicky Kalogera (Arts and Sciences), Larry Birnbaum, Fabian Bustamante and Diego Klabjan (Engineering), Joe Paris (Information Technologies), Brian Uzzi (Management), and Ramana Davuluri (Medicine).
In order to continue the momentum and increase participation across Northwestern, Luis Amaral and Adam Pah developed a Programming Bootcamp with the goal of enabling members of the Northwestern community to learn the programming skills needed to collect, process, and analyze data. The bootcamp — offered September 2014, March 2015 (Spring Break), and September 2015 — was attended by over 400 graduate students, undergraduates, postdoctoral fellows, and even staff and faculty members representing 10 Northwestern schools, including the McCormick School of Engineering, The Graduate School, Weinberg College of Arts and Sciences, Bienen School of Music, School of Education and Social Policy, School of Professional Studies, Kellogg School of Management, and School of Communication.
On the Summer of 2015, the Data Science Initiative was funded by the Office of Research for an initial period of 3 years (see Opportunities for details on activities funded). The initiative gave rise to a dense network of connections among scholars from different disciplines (see below).
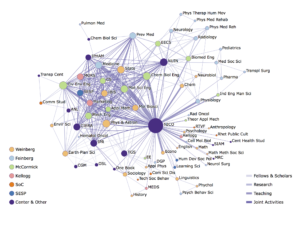